Monday, February 25, 2013
Moderate exercise
if I can do it, anybody can
Another health study in the news this morning, where they show that physical activity not only helps prevent diabetes (which we already have very good evidence for), but it also seems to greatly reduce the risk of death among people that already suffer from the disease.
In short, 40-60 year-old diabetes patients that did the equivalent of half an hour brisk walking or more every day had a 40% lower risk of dying within eight years than a control group that was sedentary. 40% is enough of an effect that it really ranks right up there with diet and medication for controlling diabetes.
One study doesn't really prove anything, and I often go on at length about that. But this fits very well with what we already know about the benefits of moderate, frequent exercise. Extraordinary claims demand extraordinary proof, but expected claims do not. And when the upside of acting if it is correct is large and the downside if it's wrong is very small, you might as well act as if it is true and not waste time waiting for more confirmation.
The study used walking as an example of activity level. But there's more and more reason to believe that walking, specifically, is especially good for your health. Low or medium-intensity exercise over long periods have better effect and less injuries than high intensity in short spurts. So walking for an hour every day is probably better for you than, say, aerobics two or three times a week. Standing and moving about instead of sitting down for hours on end may be better still.
There's many good ways to motivate yourself to do this level of exercise. I bring a camera along, and I play Ingress. Both push me to take detours, get off the train a station early, take lunchtime walks, wander through town on my days off and so on. And I often stand and work at the office — mostly for my back, so the other benefits are a bonus.
It seems to be effective — my weight, blood pressure and health exam results have all been improving over the years — but the effect is a bit lopsided. My legs and hips get lots of exercise, while my arms and shoulders get nothing. I should probably do something for my back if nothing else, but it's difficult to figure out what to do. Walking is just, well, walking, but all upper-body exercise seems to involve doing actual sports, which is something I'm just as happy to avoid.
Wednesday, February 20, 2013
Our Saccade model paper, explained
We have a paper published right now in PLoS One, covering most of what I've been up to for the past few years: The Mechanism of Saccade Motor Pattern Generation Investigated by A Large-Scale Spiking Neuron Model of The Superior Colliculus, by Jan MorĂ©n, Tomohiro Shibata and Kenji Doya. Let's take a look at what we've done and why. It's all overly long1, but stick around to the end — we've got robots!
Let's begin with the big picture. We want to understand how the brain works. Why? First, a lot of medical conditions are related to the brain in one way or another. And we won't know how to treat them or alleviate their symptoms unless we know how the brain works at different levels.
Second, animal brains are pretty good at what they do. We're good at recognizing things, find our way around, avoid danger, learn new skills and so on. A machine — a computer or robot — that could do the same would be really useful. But it's very difficult to design systems that do this kind of thing. One way is to simply copy a system that we know already works — our brains. Not copy exactly, mind you, but find out how brains do their thing, then use the same kind of methods in our robots.
Finally, of course, learning about things is what we do as a species. We need to find out about what makes us tick — and where comets come from, and how ants navigate, and why magnets work — simply because not knowing is an itch that won't go away, and we'll keep scratching that itch no matter what.
Introduction
We've learned a lot about the brain over the past century or so by looking at isolated areas. We map the structure; measure the activity as an animal behaves; stimulate neurons and see how other neurons respond; compare people with and without damage to that area and so on. Lately we've also started using computer modelling as a powerful tool: Build a model according to your theory, then see if the model produces results similar to the real thing. That's a powerful way to test your ideas, and it can also suggest new things to look for in real brains.
Looking at individual areas has been, and continues to be, spectacularly successful. But, a single brain area isn't really isolated. Every part is heavily connected to many other areas2, and any behaviour is a product of many such areas working together. As our theories and models become more and more detailed we also have to give more thought to how the area works with others, and what their ultimate functional purpose really is.
So we set out to model an area in some detail, but take into account the entire functional circuit. The area we chose is called the Superior colliculus or SC for short. It's part of the shortest, fastest visual-motor circuit in our brains.
The mammal visual system works like this: The retina in your eyes takes in images and processes them (the retina is really much more than just a passive sensor). This image data get sent to the thalamus where it's remixed, then onto the visual cortex right in the back of your head. From there, the data splits up in a number of systems for detecting objects, estimating movement and so on. It's big and complicated, and ultimately involves most of the brain.
Figure 1. A slice through the midline of the brain. The superior colliculus at the top of the midbrain, marked with a circle. The eyes are toward the left, and the location of the visual cortex is marked on the far right, though the cortex is actually wrapping around and covering all of this.
But we all have another visual system that works in parallel to this one. The retina also projects to (neuroscience-speak for "sends data to") the SC near the top of the midbrain (we actually have two collicula; one on each side). The SC in turn connects to motor neurons in the brainstem that control our eyes. You can see this loop in the image above.
This circuit generates saccades — quick eye movements from spot to spot. The top ("superficial") layer of the SC looks for sudden changes in the visual field. If it seems important, it triggers the lower ("intermediate") layer of the SC that in turn moves the eyes to that spot. That way we can quickly focus on the change, and decide if it's important. This is much simpler and much faster than the cortical vision system, so we can react quicker to sudden dangers.
This system is a simple but complete visual behaviour loop, with images to the retina as input and eye movements as output. And we know enough about the parts of this loop that we can at least start thinking about how to model the whole thing.
Figure 2. The two superior colliculuses. Left sees the right visual field and right sees the left. Top is fovea, and the numbers are degrees away from the fovea in the visual field.
The Superior Colliculus is about 5mm by 4mm, and around 2mm thick in primates. It is retinotopic, that is, it's a map of the visual input. We have one SC on each side, and in primates each one maps the opposite half of the visual field. The fovea, our point of focus, is at the top. The vertical midline goes along the edges, with up along the inner edge and down along the outer edge. Straight left and right goes down along the center of each surface.
The whole map is bent and skewed so that areas close to the center — where we have good vision — get lots of space, while the blurry areas towards the corner of our eyes get very little. As you can see, the visual field within ten degrees from our fovea occupies more than a third of the entire surface, and the first 20 degrees occupies more than half. The remaining 60-70 degrees of our visual field is all crammed in the remaining space.
When something suddenly appears in the visual field, the retina will detect it and send it on to the superficial SC. Neurons in that point of the map will activate. If the activation is strong enough, and if there's nothing else occupying our attention, that will in turn activate a neuron circuit at the same point in the intermediate layer.
That neuron circuit will move our eyes to that spot by activating motoneurons in the brainstem that in turn connect to the muscles that move our eyes. The details are controversial, but there's a fair amount of evidence that the output from the SC doesn't just encode a place, but actually tells the eyes how fast move over time. The stronger the output the faster the eyes will move, and if you disrupt the output from the SC, the eye movement changes along with the disruption.
Many researchers believe the SC only points out the position, and doesn't specify the eye movements. They're not stupid, unskilled or dishonest, and neither are those that, like us, think it does. We genuinely don't yet know which is right; and it may be that the answer is different for different animals. One day we may know for sure, but until then it is the cause of a lot of heated arguments.
The major point of the superior colliculus is that it forms the boundary between perception on the superficial side, and action on the intermediate side. In most perception-action systems in the brain, this step from one side to another is very complex and hard to study, but here it is (comparatively) simple and clear. That makes this a great target for study.
The Model
Our model focuses on the saccade-generating circuit in the intermediate layer of the SC. We use the NEST neural simulator, and as the model is fairly big and computationally heavy we've used the K supercomputer and the RICC large-scale cluster for most of our simulations3. The model itself uses around 100 000 model neurons; medium to large-scale but not really big.
One question we want to answer is how, exactly, the local circuit in the SC generates the output that drives the eyes. This output — spikes generated by so-called burst neurons — needs to have a specific, well-defined shape over time in order to create the right eye movement.
Burst neurons get their name because they output bursts of spikes — that is, lots of spikes in a short time. When burst neurons get activated by a combination of two different inputs, they will send a burst of spikes down to the eye motoneurons and on to the eye muscles.
But this output is also sent to an integrator, a spike counter, which seems to reside in a small area right next to the SC. As this spike counter receives more and more spikes, it will output more spikes in turn back to the burst neurons. This output inhibits the burst neurons, so their activity gradually drops over time. This profile (you can see it two figures down), with a rapid burst followed by a gradual drop, is what we need to get correct eye movements. This circuit is illustrated in figure 3A, below:
Figure 3. A, to the left, is the burst circuit. B, to the right, is the buildup circuit. cMRF stands for "central Mesencephalic Reticular Formation" and is our proposed location for the integrator circuit.
The other question is about buildup neurons, another neuron type in the same area. Before and during a saccade, the activity of buildup neurons will gradually increase and spread through the SC. Imagine spilling ink at the target point, then see the stain spread across the surface, more towards the fovea than in the other direction. The question is why the buildup neurons do this.
There's been a number of theories about why many animals have this spreading in the SC. Most of them assume that it has to do with tracking the eye position or stopping the eye movements at the right time. Perhaps the center of spreading or the edge of the spread tracks the current position of the eyes, or perhaps the eye is stops when the spread reaches the top edge of the SC.
But no theory seems to account for all facts — and in fact, at this level of detail, different animals might do things differently. A cat's paw, for instance, with soft pads and retractable claws, is very different from a horse's hard, solid hoof or a rat's grippy finger-like feet. We shouldn't be surprised if their brains also differs in detail. It's not at all impossible that some of these theories could be correct for one animal but not for another.
Our idea is that the amount of buildup neuron activity, but not the spreading itself, is what's important. The spreading is just a reliable way to increase total activity, and the area it covers is largely irrelevant. Once the activity reaches a certain level, it will trigger inhibitory neurons that in turn shut down the SC and ends the saccade.
Our proposed circuit is shown in figure 3B above. Our buildup neurons are connected to each other. If one starts firing, it will prod nearby ones to also start firing and so on in turn, until they all fire. But they are mingled with interneurons that inhibits the buildup neurons. This lets them be active, but not spread the activity far.
Now, remember the spike integrator I mentioned above? It inhibits these interneurons, and lets the buildup neuron activity spread. The more spikes the spike counter has received, the more it inhibits those interneurons, and the more the buildup neuron activity will spread. And as I wrote above, that spread ultimately activates inhibitory neurons that ends the saccade. Figure B above shows this circuit, and below is the complete circuit we use in the model.
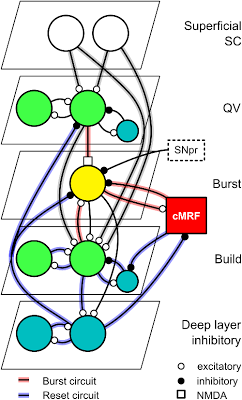
So far, so good. We have a model. But we still need to tune it using real data, and we need to interpret the output.
As I said, the burst neurons need to generate a specific output profile. So what we do is take published monkey burst neuron data, then tune two connections — one of the inputs to the burst neuron, and the feedback from the spike counter.
The actual tuning process is a bit involved, but briefly, we look at measured peak burst rate and burst time in monkey burst neurons (from Goossens and van Opstal4) for a few different distance saccades, then tune the input strengths to our burst neurons so their peak and time matches. The circuits for a particular saccade distance on the surface all use the same input strength parameters, and circuits for in-between distances get in-between values.
Figure 5. Monkey burst neuron data is to the left. As you can see, short saccades have a high peak but short duration. Longer saccades have lower peaks and longer durations. The total number of spikes they send is roughly constant for all saccades. To the right is our model burst neurons outputs after tuning.
The output from the model is a bunch of spikes from the burst neurons. We know that these spikes are supposed to drive the eye muscles, but we need to figure out how to map from spikes to movement. As it turns out, Tabareau and Berthoz5 have already figured out how the spikes from each point on the SC should be weighted to generate eye movement. If we use the same weights, we can see what kind of eye movement our model would create.
What we look for is the so-called main sequence. Eye movements are very stereotypical, and saccades always move in the same way. Specifically, the top speed of the eye ball will increase with longer saccades, but the increase will drop off. The saccade time on the other hand, will increase linearly with distance. And finally, diagonal saccades will have lower peak speed and longer duration than vertical or horizontal ones.
Saccade profiles. As you can see we get sub-linear peak speed and linear time. Inset is human saccade data for comparison. To the right we show that we also get lower peak speed and longer duration for diagonal saccades than for horizontal or vertical saccades with the same component-wise distance.
What have we learned? First, we have not proved that this is the way the intermediate SC actually works. After all, this is still an incomplete model of the real circuit, and missing quite a lot of things. What we do show, however, is that the area feasibly could work like this, given what we know about it.
We also suggest that the point of the buildup-neuron spreading activation may in fact not be the spreading itself, but just the gradual total activity buildup in the area. Again, it's a suggestion, not proof.
And in general, we make use of a lot of experimental data, some that is solidly grounded, some that is tentative and uncertain. And this model shows that the data we used is at least not incompatible with the SC directly determining the eye movement profile. We don't show the data is right, but we do show that it could be right.6
This is one of the strengths of computer models: they can show quite clearly when an idea is wrong and won't work. In fact, that often becomes clear long before you actually have a complete, runnable model. The very act of formalising your ideas, putting them in the explicit form of equations or a computer program, will expose any hidden assumptions, inconsistencies and other problems with your ideas. The work really forces you to understand the system in a way you would not otherwise, and that helps generate new ideas and approaches; our idea of total activity being important came about in this way.
I would even go as far as saying most of the value of a model comes from developing it, not running it. That's where all the effort is spent, and that's where you get most of your insights. I could imagine spending months on building a good model, and never actually run the finished version for real.
A model doesn't have to be finished, and it also doesn't have to be complete in any sense to be useful. In our case, we have completely neglected several important parts. The fovea, for instance, is actually different than the rest of the SC. It is active while we fixate and causes the eyes to do very small jumps back and forth. And the SC doesn't just do eye movements; for large saccades it actually triggers head movements too.
We also ignore the cerebellum, which is critical for tuning the eye movements, and we have none of the brainstem circuits that translate the movement commands into muscle activation. We just assume that the output is weighted correctly. We lack a retina and most of the top layers, and instead we just simulate the kind of input we think this area would get from them.
That is all fine. One point of a model is to abstract away anything that is not directly relevant. Our aim for the paper was to explore how the circuit in the SC actually generates eye movement commands. But this has all been about one part of the SC. What about considering the whole circuit that I was talking about in the beginning? And what about robots? Our paper stops here, but we didn't stop with the paper. We still wanted to try to create the whole loop in some way.
Now, With More Robot!
![]() |
CBi robot. © ATR (but taken by me) |
We had two problems to overcome. First, to do this the model would have to work fast. The eye movement can't take seconds; it would have to at least look realistic. We'd also need two models, one each for the left and right visual fields, and connect them. But that would double the size of the model, making it even slower. How slow was it? My estimate was that we'd need about 8000 cores to run the original model in real time. It's not easy getting exclusive use of that kind of resource for hours or days at a time. Besides, fewer resources means fewer problems and faster development.
Instead, we scaled down the original model. The problem is that with fewer neurons the model becomes noisier and more error-prone, so the accuracy drops. We managed to scale it down to around 1/6 of the original size, and also employed a couple of other tricks to speed it up. In the end, we estimated about 500-800 cores would get us to real time, and 256 cores (our regular allocation) would be fast enough to try things out.
The second problem was the location. We use the CBi humanoid robot that I've worked with before. The robot is at nearby ATR in south Kyoto. For the robot we use the RICC large-scale cluster at RIKEN in Wako-shi north of Tokyo. Neither robot nor cluster is portable. The solution is to connect them over the internet. This slows down communication but should still be workable.
This is the overall organization. We have the robot at ATR, and the cluster at RIKEN in Wako. At ATR we grab images from the robot, and implement a very simple saliency map that picks the most significant point in the image. This is sent over the net through a VPN to the front-end of the cluster, then on to an input process running on the cluster itself.
That process figures out what input the SC would get from a visual input like this, and uses a library called MUSIC to send input spikes to the model. The model does its thing, using most nodes we have available, and the output spikes are sent on to an output process, again using MUSIC for the communication.
The output spikes are weighted according to their origin, packaged up and sent back to ATR via the front-end. At ATR the spikes are scaled, and used directly to move the robot eyes. Each spike simply moves the eye some fraction of a degree in the horizontal and vertical direction.
Here's a video clip of the system in action. Top left is the robot head. Top right is the view from an external video camera. Bottom right is the view from the robot eye itself. And bottom left is a plot of the eye gaze direction over time. it tries to saccade to the ball.
We use 256 cores in total in this video clip. That gives us a speed about half real time, but as you can see that is still fast enough to seem realistic to observers.
The communication time from ATR, into the cluster at RIKEN, then back to ATR is around 110 milliseconds. That's not too bad when you consider the distance; the use of layers of VPNs; and the multiple steps on the cluster side. We could probably cut the time in half, and even less if we could have computer and robot in the same local area.
This is a tech demo, not science. That is, we did this to demonstrate our model; to see if we can connect it to the real world over large distance using a robot; and see how well this all works in practice. It is not meant to be any kind of scientific result, though you can use a similar set-up for research. It could be useful to evaluate large-scale models in a real-world setting and for using behaving robots in experiments.
It works pretty well. The performance is so-so — we scale down the model and that makes it miss the target position pretty badly now and again. And we only discovered that the other eye mirrors the active eye movement after the experiment, when we started editing the video clips. But overall I'm happy and surprised at how well it performs.
As our models get more detailed and complex, they will need more realistic inputs and outputs. I believe that with further refinement this kind of set-up can be a practical way to test computationally heavy models in real-world settings.
---
#1 If you think this is long, you should have seen the early drafts.
I really don't know how much I should explain here, and what can I assume that you know or can find out on your own. For instance, I cut out everything about real neurons and about the simple neuron model we use in this paper. Should I have kept it in or is it better left out? I have no idea. What I do know is that I often get too wordy, so I remove things when in doubt.
I really don't know how much I should explain here, and what can I assume that you know or can find out on your own. For instance, I cut out everything about real neurons and about the simple neuron model we use in this paper. Should I have kept it in or is it better left out? I have no idea. What I do know is that I often get too wordy, so I remove things when in doubt.
#2 This can really play a trick on you if you're not careful. If you take a brain region — thalamus, say, or Hippocampus — and draw all the connections to and from it, you'll see it's connected to stuff all over the brain. It's easy to conclude your area is a nexus of some sort and especially important for all sorts of things. But most areas are heavily interconnected, and would show the same kind of wide influence.
#3 This is purely informational; I don't get a commission if you order a supercomputer through these links. I sure wish I did, though.
#4 Linear ensemble-coding in midbrain superior colliculus specifies the saccade kinematics. This paper is really the main inspiration for the model we've done here.
#5 Geometry of the superior colliculus mapping and efficient oculomotor computation. Good paper, and the math is not difficult, but there is a fair amount of it.
#6 A lot of science is like this: you don't get a single piece of definite proof one way or another. Instead you slowly scrape together a pile of evidence showing that no other explanation will fit with what we know. Any one piece of evidence is often uncertain, ambiguous and tentative, and may contradict other pieces. It's only in the aggregate that we can get a clear picture of how things actually work.
The next time you see a headline about some new research result — especially in medicine — please remember that a single paper and a single result is almost worthless by itself, and it only matters as a small part of the slow-growing pile of accumulating evidence. This is also why that dream of "overturning all what we know in a single stroke!" that cranks are so fond of is so completely off: no single paper, and no single result can really ever do that.
The next time you see a headline about some new research result — especially in medicine — please remember that a single paper and a single result is almost worthless by itself, and it only matters as a small part of the slow-growing pile of accumulating evidence. This is also why that dream of "overturning all what we know in a single stroke!" that cranks are so fond of is so completely off: no single paper, and no single result can really ever do that.
Monday, February 18, 2013
Research Papers and Their Audience
— how to share our results
We have a new paper published on Wednesday morning, and anybody will be able to download it or read it online. I'll put up a post about the research itself once it goes live, but before I do that I thought I'd say a bit about papers in general.
Our paper is Open Access, which means anybody can read it for free. This is a good thing; you and I have all paid for the research through our taxes so we should all get to read results. That doesn't mean that we all can. Research papers are not written for a general audience. In fact, they're not even written for researchers in general1. We really write our papers for other people working in the same, narrow subfield, and if you don't already know most of the background and jargon it will often be impossible to follow.
Why do we do this? We're not trying to make it difficult for the public to read, but making it very easy for other specialists to do so. The structure and the language of a paper is tightly controlled. If you are familiar with this structure you can decide if a paper is relevant to you in a matter of seconds, and find the essential points in just a few more moments.
I usually have from 30 to 100 new papers waiting for me each morning. I normally go through them in less than half an hour, and that includes reading the relevant ones in more detail. If I couldn't sort out the important ones quickly and accurately, I would spend all morning doing nothing else. The research paper — odd structure, stilted language, impenetrable jargon and all — is invaluable for this.
So how do we share results widely then? It used to be through science journalists that translated research results for the public. Some journalists and some media do truly excellent work; others, not so much. A lot of research is covered badly or superficially, and most research never get any public coverage at all. Far too often, a single ambiguous result gets oversimplified and blown up into a three-word headline ("Gerbils Cause Cancer!"), with no background, no nuance and no warning that it's nothing definite. When more data comes in showing that gerbils in fact don't cause cancer, the same journalists and newspapers will be completely silent.
But today we have the net. Anyone that is interested can look at the original research papers (if they're Open Access), read science-oriented blogs — Ed Youngs Not Exactly Rocket Science and Carl Zimmer's The Loom are two great places to start — and even ask the researchers directly.
Of course, there's a lot of crappy coverage out there — pseudo-science, religious nonsense, lots of people pushing an economic or ideological agenda — but then, most traditional coverage can be just as biased and just as awful. Just search for, say, homeopathy and despair at the amount of gullible, fawning coverage in established media and amateur blogs alike.
We can combat this, in a way that was never possible before the net. The internet is a cesspool, but unlike newspapers or TV it's a mostly transparent one. The good coverage is out there along with all the bad, and we can sort it out with a bit of work and thought on our own part. One way we specialists can help is by making our papers Open Access. Research papers are the primary sources after all. If a newspaper claims that "X causes cancer!" you can go look at the paper itself — or go read the blog of somebody like Ed Young who did — and see if the breathless coverage really is accurate; true but overblown; or a useless pile of link-bait making stuff up out of thin air.
We can also help by being more visible online. The research paper is our primary way to share our results — but it doesn't have to be the only one. Many researchers don't have the time nor the inclination to run a blog or spend time on social sites. Many of those that do, use blogs and social media to relax from work, not add to it. But when we can, we should post about our research directly as well. We, as a group, really need to be more visible, and not give up the stage completely to the kooks, the religious zealots, the political ideologues and the con artists that all have emotional or economic reasons to oppose good science.
So come Wednesday I'll do my bit with a post about our new paper, explain what we've done and try to give you some of the background that the paper itself doesn't have. Don't miss it; there will be robots!
Our paper is Open Access, which means anybody can read it for free. This is a good thing; you and I have all paid for the research through our taxes so we should all get to read results. That doesn't mean that we all can. Research papers are not written for a general audience. In fact, they're not even written for researchers in general1. We really write our papers for other people working in the same, narrow subfield, and if you don't already know most of the background and jargon it will often be impossible to follow.
Why do we do this? We're not trying to make it difficult for the public to read, but making it very easy for other specialists to do so. The structure and the language of a paper is tightly controlled. If you are familiar with this structure you can decide if a paper is relevant to you in a matter of seconds, and find the essential points in just a few more moments.
I usually have from 30 to 100 new papers waiting for me each morning. I normally go through them in less than half an hour, and that includes reading the relevant ones in more detail. If I couldn't sort out the important ones quickly and accurately, I would spend all morning doing nothing else. The research paper — odd structure, stilted language, impenetrable jargon and all — is invaluable for this.
So how do we share results widely then? It used to be through science journalists that translated research results for the public. Some journalists and some media do truly excellent work; others, not so much. A lot of research is covered badly or superficially, and most research never get any public coverage at all. Far too often, a single ambiguous result gets oversimplified and blown up into a three-word headline ("Gerbils Cause Cancer!"), with no background, no nuance and no warning that it's nothing definite. When more data comes in showing that gerbils in fact don't cause cancer, the same journalists and newspapers will be completely silent.
But today we have the net. Anyone that is interested can look at the original research papers (if they're Open Access), read science-oriented blogs — Ed Youngs Not Exactly Rocket Science and Carl Zimmer's The Loom are two great places to start — and even ask the researchers directly.
Of course, there's a lot of crappy coverage out there — pseudo-science, religious nonsense, lots of people pushing an economic or ideological agenda — but then, most traditional coverage can be just as biased and just as awful. Just search for, say, homeopathy and despair at the amount of gullible, fawning coverage in established media and amateur blogs alike.
We can combat this, in a way that was never possible before the net. The internet is a cesspool, but unlike newspapers or TV it's a mostly transparent one. The good coverage is out there along with all the bad, and we can sort it out with a bit of work and thought on our own part. One way we specialists can help is by making our papers Open Access. Research papers are the primary sources after all. If a newspaper claims that "X causes cancer!" you can go look at the paper itself — or go read the blog of somebody like Ed Young who did — and see if the breathless coverage really is accurate; true but overblown; or a useless pile of link-bait making stuff up out of thin air.
We can also help by being more visible online. The research paper is our primary way to share our results — but it doesn't have to be the only one. Many researchers don't have the time nor the inclination to run a blog or spend time on social sites. Many of those that do, use blogs and social media to relax from work, not add to it. But when we can, we should post about our research directly as well. We, as a group, really need to be more visible, and not give up the stage completely to the kooks, the religious zealots, the political ideologues and the con artists that all have emotional or economic reasons to oppose good science.
So come Wednesday I'll do my bit with a post about our new paper, explain what we've done and try to give you some of the background that the paper itself doesn't have. Don't miss it; there will be robots!
#1 I read a short paper on paleoceanography — estimating the age of ice cores and that sort of thing — just the other day. The method parts I was asked to comment on were fine, but the hardcore palaeoclimatology discussion could have been written in Sanskrit as far as I was concerned. I have plenty of general science background, but I just don't have all the specialist knowledge to make sense of the details.
Thursday, February 14, 2013
Valentine's Day
It's valentine's day, and this year Ritsuko got me this:
Camel's milk chocolate, from Al Nassma. Three kinds: Macadamia-Orange, Arabia — that's spiced with aniseed, cardamom, coriander and cinnamon — and straight 70% cacao. I've only tried the Arabia so far, and it's very tasty. The spices are clear but not overpowering and work really well with the cacao flavour. Looking forward to try the other two kinds as well!
Camel's milk chocolate, from Al Nassma. Three kinds: Macadamia-Orange, Arabia — that's spiced with aniseed, cardamom, coriander and cinnamon — and straight 70% cacao. I've only tried the Arabia so far, and it's very tasty. The spices are clear but not overpowering and work really well with the cacao flavour. Looking forward to try the other two kinds as well!
Wednesday, February 6, 2013
JLPT
Once again I did the Japanese Proficiency Test, and I just barely missed. With 97/180 points it's three points better than last year and only three points from a passing grade. This is a better result than I expected.
Work and private life forced me to drop my Japanese studies altogether for six months, and starting a new job gave me no time to study for the test. I'm just happy I didn't do worse than previous years. Next year perhaps.
Work and private life forced me to drop my Japanese studies altogether for six months, and starting a new job gave me no time to study for the test. I'm just happy I didn't do worse than previous years. Next year perhaps.
Monday, February 4, 2013
UQ WiMAX Router, a Review
We've started using UQ WiMAX for mobile connections lately. It promises to give you unlimited fixed-net speeds anywhere, to any wifi-equipped device you own. I've posted a review of it at Japan Mobile Tech. Go check it out!
Subscribe to:
Posts (Atom)